Leon Bungert, Prof. Dr.
Prof. Dr. Leon Bungert
W2 Professor with Tenure Track to W3
Professorship for Mathematics III (Mathematics of Machine Learning)
Emil-Fischer-Straße 40
97074
Würzburg
Building:
Mathematik Ost (40)
Room:
01.008
Phone:
+49 931 31-82849
Fax:
+49 931 31-81492
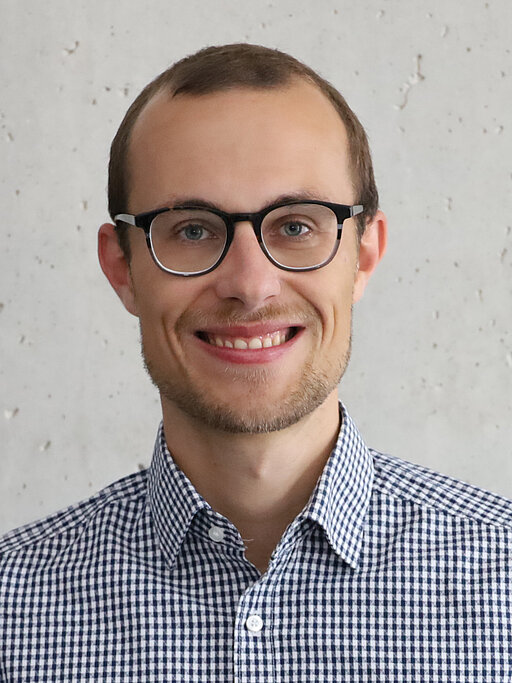
Professor (Tenure Track) at the University of Würzburg since 2023
Previous Positions:
- Junior Research Group Leader at the Technical University of Berlin (2023)
- Postdoctoral Researcher at the Hausdorff Center for Mathematics, University of Bonn (2021 - 2023)
- Postdoctoral Researcher at the University of Erlangen-Nürnberg (2020 - 2021)
Education:
- Ph.D. (summa cum laude) from the University of Erlangen-Nürnberg (2020), title of the thesis: "Nonlinear spectral theory with variational methods"
- M.Sc. in Mathematics from the University of Erlangen-Nürnberg (2017)
- B.Sc. in Mathematics from the University of Erlangen-Nürnberg (2016)
-
It begins with a boundary: A geometric view on probabilistically robust learninghttps://arxiv.org/abs/2305.18779 (2023)
- [ URL ]
-
The convergence rate of $p$-harmonic to infinity-harmonic functionshttps://arxiv.org/abs/2302.08462 (2023)
- [ URL ]
-
Polarized consensus-based dynamics for optimization and samplinghttps://arxiv.org/abs/2211.05238 (2022)
- [ URL ]
-
Ratio convergence rates for Euclidean first-passage percolation: Applications to the graph infinity Laplacianhttps://arxiv.org/abs/2210.09023 (2022)
- [ URL ]
-
Gamma-convergence of a nonlocal perimeter arising in adversarial machine learninghttps://arxiv.org/abs/2211.15223 (2022)
- [ URL ]
-
Neural Architecture Search via Bregman Iterationshttps://arxiv.org/abs/2106.02479 (2021)
- [ URL ]
-
The lion in the attic -- A resolution of the Borel--Kolmogorov paradox(2020)
-
The infinity Laplacian eigenvalue problem: reformulation and a numerical schemehttps://arxiv.org/abs/2004.08127 (2020)
- [ URL ]
-
The geometry of adversarial training in binary classificationInformation and Inference: A Journal of the IMA 12, 921-968 (2023)
- [ DOI ]
-
The inhomogeneous $p$-Laplacian equation with Neumann boundary conditions in the limit $ ptoinfty$Advances in Continuous and Discrete Models 2023, 1-17 (2023)
- [ DOI ]
-
Uniform convergence rates for Lipschitz learning on graphsIMA Journal of Numerical Analysis 43, 2445-2495 (2023)
- [ DOI ]
-
Complete Deterministic Dynamics and Spectral Decomposition of the Linear Ensemble Kalman InversionSIAM/ASA Journal on Uncertainty Quantification (2023)
- [ DOI ]
-
Eigenvalue problems in $mathrmL^infty$: optimality conditions, duality, and relations with optimal transportCommunications of the American Mathematical Society 2, 345–373 (2022)
- [ DOI ]
-
Continuum Limit of Lipschitz Learning on GraphsFoundations of Computational Mathematics (2022)
- [ DOI ]
-
A Bregman Learning Framework for Sparse Neural NetworksJournal of Machine Learning Research 23, 1-43 (2022)
-
Nonlinear power method for computing eigenvectors of proximal operators and neural networksSIAM Journal on Imaging Sciences 14, 1114-1148 (2021)
- [ DOI ]
-
Nonlinear spectral decompositions by gradient flows of one-homogeneous functionalsAnalysis & PDE 14, 823-860 (2021)
- [ DOI ]
-
Structural analysis of an $L$-infinity variational problem and relations to distance functionsPure and Applied Analysis 2, 703–738 (2020)
- [ DOI ]
-
Localization of Passive 3-D Coils as an Inverse Problem: Theoretical Analysis and a Numerical MethodIEEE Transactions on Magnetics 56, 1-10 (2020)
- [ DOI ]
-
Asymptotic profiles of nonlinear homogeneous evolution equations of gradient flow typeJournal of Evolution Equations 20, 1061-1092 (2020)
- [ DOI ]
-
Variational regularisation for inverse problems with imperfect forward operators and general noise modelsInverse Problems 36, 125014 (2020)
- [ DOI ]
-
Robust Image Reconstruction with Misaligned Structural InformationIEEE Access 8, 222944-222955 (2020)
- [ DOI ]
-
Solution paths of variational regularization methods for inverse problemsInverse Problems 35, 105012 (2019)
- [ DOI ]
-
Robust Blind Image Fusion for Misaligned Hyperspectral Imaging DataPAMM 18, e201800033 (2018)
- [ DOI ]
-
Blind image fusion for hyperspectral imaging with the directional total variationInverse Problems 34, 044003 (2018)
- [ DOI ]
-
Comparison of two local discontinuous Galerkin formulations for the subjective surfaces problemComputing and Visualization in Science 18, 193-202 (2018)
- [ DOI ]
-
A discontinuous Galerkin method for the subjective surfaces problemJournal of Mathematical Imaging and Vision 58, 147-161 (2017)
- [ DOI ]
-
Improving Robustness against Real-World and Worst-Case Distribution Shifts through Decision Region QuantificationIn: Chaudhuri, K., Jegelka, S., Song, L., Szepesvari, C., Niu, G., and Sabato, S. (eds.) Proceedings of the 39th International Conference on Machine Learning. pp. 19434-19449. PMLR (2022)
-
Chapter 13 - Gradient flows and nonlinear power methods for the computation of nonlinear eigenfunctionsIn: Trélat, E. and Zuazua, E. (eds.) Numerical Control: Part A. pp. 427-465. Elsevier (2022)
- [ DOI ]
-
Identifying untrustworthy predictions in neural networks by geometric gradient analysisIn: de Campos, C. and Maathuis, M. H. (eds.) Proceedings of the Thirty-Seventh Conference on Uncertainty in Artificial Intelligence. pp. 854-864. PMLR (2021)
-
CLIP: Cheap Lipschitz Training of Neural NetworksIn: Elmoataz, A., Fadili, J., Quéau, Y., Rabin, J., and Simon, L. (eds.) Scale Space and Variational Methods in Computer Vision. pp. 307-319. Springer International Publishing, Cham (2021)
- [ DOI ]
-
Computing nonlinear eigenfunctions via gradient flow extinctionIn: International Conference on Scale Space and Variational Methods in Computer Vision. pp. 291-302. Springer (2019)
- [ DOI ]